Author details
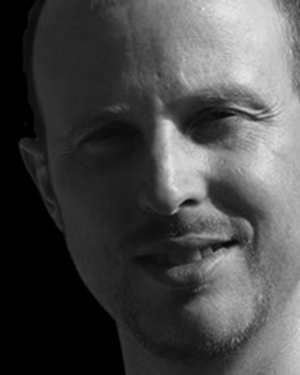
Roland Badeau
Also published under: R. Badeau
Affiliation
LTCI
Télécom Paris
Institut Polytechnique de Paris, Palaiseau, France
Biography
Roland Badeau (Senior Member, IEEE) received the Ph.D. degree from the École Nationale Supérieure des Télécommunications, Paris, in 2005, and the Habilitation degree from the Université Pierre et Marie Curie, Paris VI, in 2010. He is currently a Full Professor with the Signal, Statistics, and Machine Learning (S2A) Group of the Image, Data, Signal (IDS) Department, Tcom Paris, France. He is the co-author of more than 30 journal papers, more than 100 international conference papers, and four patents. His research interests include statistical modeling of non-stationary signals (including adaptive high resolution spectral analysis and Bayesian extensions to NMF), with applications to audio and music (source separation, denoising, dereverberat... Author's Published Works