Author details
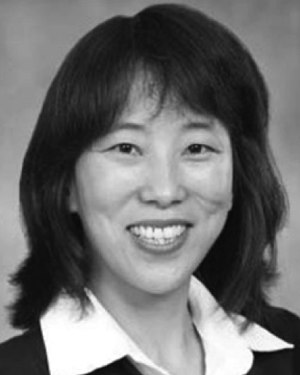
Jun Li
Affiliation
China University of Geosciencies, Wuhan, China
Biography
Jun Li (Fellow, IEEE) received the B.S. degree in geographic information systems from Hunan Normal University, Changsha, China, in 2004, the M.E. degree in remote sensing from Peking University, Beijing, China, in 2007, and the Ph.D. degree in electrical engineering from the Instituto de Telecomunicaç oes, Instituto Superior Técnico (IST), Universidade Técnica de Lisboa, Lisbon, Portugal, in 2011.,She is currently a Full Professor with the School of Computer Science, China University of Geosciences, Wuhan, China. Her main research interests comprise remotely sensed hyperspectral image analysis, signal processing, supervised and unsupervised learning, and active learning.,Prof. Li is the Editor-in-Chief of IEEE Journal of Selected Topics in ... Author's Published Works