Author details
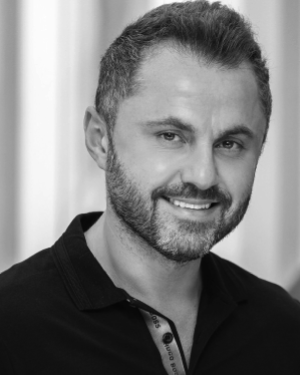
Azzam Mourad
Also published under: A. Mourad
Affiliation
Department of Computer Science
KU 6G Research Center
Khalifa University, Abu Dhabi, UAE
Artificial Intelligence and Cyber Systems Research Center
Lebanese American University
Beirut, Lebanon
Biography
Azzam Mourad (Senior Member, IEEE) received the M.Sc. degree in CS from Laval University, Canada, in 2003, and the Ph.D. degree in ECE from Concordia University, Canada, in 2008. He is currently a Professor of Computer Science and the Founding Director of the Cyber Security Systems and Applied AI Research Center with Lebanese American University, a Visiting Professor of Computer Science with New York University Abu Dhabi, and an Affiliate Professor with the Software Engineering and IT Department, Ecole de Technologie Superieure, Montreal, Canada. His research interests include cyber security, federated machine learning, network and service optimization and management targeting IoT and IoV, cloud/fog/edge computing, and vehicular and mobile ... Author's Published Works