Author details
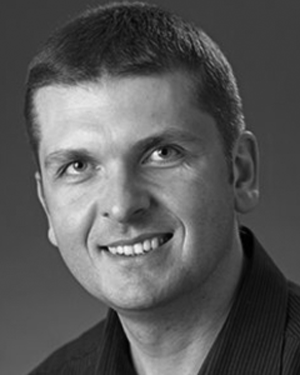
Rafal A. Angryk
Also published under: Rafal Angryk, R. A. Angryk
Affiliation
Dept. of Computer Science
Georgia State University
Atlanta, GA, USA
Biography
Rafal Angryk is an associate Professor in the Computer Science Department at Montana State University. He received his M.S. and Ph.D. in computer science degree from Tulane University, New Orleans. His current research interests lie in the areas of Data Mining, Databases (Spatial Databases, Fuzzy Database Models), Distributed Systems (Mobile Agents Technology, Distributed Databases), and Artificial Intelligence (Fuzzy Expert Systems, Neural Networks).(Based on document published on 11 April 2011). Author's Published Works